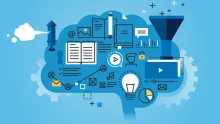
Generative AI: How does it affect the enterprise?
Blog: The Enterprise Project - Enterprise Technology
We are in the early days of generative AI, and there’s a gold rush to gain position and prominence in the sector as it takes off. But with this rush to implementation across a bewildering range of use cases come associated risks. Get artificial intelligence (AI) right, and it can be an incredibly creative, labor-saving, and efficiency-improving solution. Employ it badly, and you risk social, financial, and even legal consequences.
What are the challenges of AI for enterprises?
Organizations face several significant challenges in their AI enterprise projects. First, there’s the difficulty of predicting their value or likely success, given the unpredictability of AI outputs. Second, it can be difficult to demonstrate ROI on AI efforts since so much of the field is speculative in this experimental phase.
Third, there are risks associated with compliance, privacy, and bias when AI is set loose on large datasets or the internet itself. The old computer programming adage of GIGO (garbage in, garbage out) applies. Depending on the inputs, AI can produce spectacular prose, or text peppered with terrible factual errors and inconsistencies, as ChatGPT’s detractors have pointed out.
In addition, to make AI work, it needs to be trained on enormous datasets to generate the best responses to unpredictable situations.
Organizations need to invest in the right AI infrastructure, secure storage, and support in a period of great uncertainty. There’s a lot of talk these days about exactly how to regulate the use of AI, with artists most recently voicing concern about the aesthetics of AI-generated illustration and their intellectual property being mined to generate imitative works for which they are not recognized or paid.
[ Related read: How the democratization of AI impact enterprise IT. ]
Added to this list of concerns are worries about unintended consequences, a lack of consensus over best practices, and a feeling of over-reliance on technology that isn’t yet fully understood (and whose algorithms remain opaque even to their creators).
AI as grand disruptor–is this a good thing?
When people aren’t fretting over its implications, generative AI is often considered an impressive disruptor within modern enterprises. Its presence is felt everywhere: in market research, customer service, sales, education, and even the delicate business of client liaison. As a tool for working out what customers want and designing exactly that, it is revolutionizing innovation, design, and delivery.
Two areas of great innovation are synthetic data and autonomous enterprise. The former describes the creation of wholly invented but typical datasets that can be used to test new products. Data synthesis can also create unlikely, but not impossible, scenarios to test how AI-driven systems would deal with such occurrences.
Consider the testing of autonomous vehicles, for instance. It’s infinitely easier and better to simulate a deer running across a freeway at rush hour than it is to run any kind of real-life test. Program in a thousand such scenarios with subtle variations (such as a herd of deer, an especially slow or fast deer, or animals coming from multiple angles), and you’ll soon generate highly effective avoidance systems, the theory goes.
Data scarcity is probably the biggest hurdle to overcome in improving AI. Many industry-specific or domain-specific AI models require large amounts of data to inform machine learning, but such data can be scarce or difficult to obtain.
The problem is compounded by a shortage of data scientists with domain expertise, making it difficult to develop reliable AI models. Additionally, AI requires data that is well-validated and complex. If the data pool does not match the AI’s existing data models, this can lead to performance failures.
How can generative AI help?
Generative AI can help transform enterprise projects by providing data science teams with the ability to create synthetic data and produce successful algorithmic models. It can also be used to create high-value artifacts such as images, video, text, code, and even designs and schematics.
Rather than threatening to put human artists, coders, and designers out of work, generative AI can bridge the gap between human creativity and technological innovation. It can also be used to develop novel deep-learning algorithms for diagnostically challenging tasks.
[Related read: 9 ways ChatGPT will help CIOs. ]
This is having powerful impacts in the field of cancer diagnostics, for instance, with AI-driven visual algorithms achieving a success rate equal to, or better than, experienced radiologists in tumor detection. AI can even predict tumor regrowth with startling accuracy.
Can generative AI help deliver innovative enterprise projects?
The short answer is yes… if it’s used correctly.
AI will inevitably transform how businesses and individuals create digital solutions. The second area of innovation mentioned earlier is the notion of the autonomous enterprise. Companies are employing generative AI to design, maintain, and monitor intricate systems with minimal manual effort. Now there are moves to create fully or largely autonomous business models where customers interact with AI-powered assistants or chatbots, and the entire delivery and support mechanism is automated.
For the moment, however, to use generative AI effectively, you still need human input at the start and human validation for the outputs. And let’s not forget the complex ethical implications of all that autonomy, from IP theft to dangerous deep fakes. Organizations need to adopt AI without losing sight of the human consequences and costs of all that radical innovation.
The rapid growth of generative AI has both positive and negative implications for enterprises. Companies must invest in the right AI infrastructure and ensure human validation for the outputs. Generative AI’s potential is endless, and it can bridge the gap between human creativity and technological innovation when done correctly.
[ Want best practices for AI workloads? Get the eBook: Top considerations for building a production-ready AI/ML environment. ]
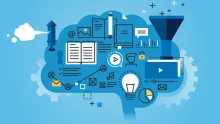